WiForAgri
What was the main challenge, who are you helping
Smart Agriculture and IoT applications dedicated to the viticultural world have begun to emerge in EU for about 10 years thanks to the enabling technology (the possibility of creating low-cost embedded systems) and thanks to the spread of Internet access even in rural areas (thinks about 4G and LPWAN i.e. LoRa, Sigfox et al.). The main expectation on these IoT smart-agriculture applications concerns the possibility of optimizing (and reducing) some strategic production inputs such as chemical phytosanitary treatments. However, these first IoT applications, although they create great expectations in the agriculture sector, do not always succeed in their intent. One of the critical points concerns the availability and reliability of the disease prediction-models (row data from sensors are not enough to archive benefits) and their adaptability (tuning an training) to the different cases of application that exist in agricultural and viticultural contexts (variability of soils, plant genetics, microclimates). Consequently, the initial investment is not always perceived by the farmer as convenient and the market has so far developed less than initial expectations. This scenario highlights the need (and the lack on the market) for reliable, customizable and specialized IoT integrated solutions, including specific sensors (for each crop) and trainable models (for each target disease).
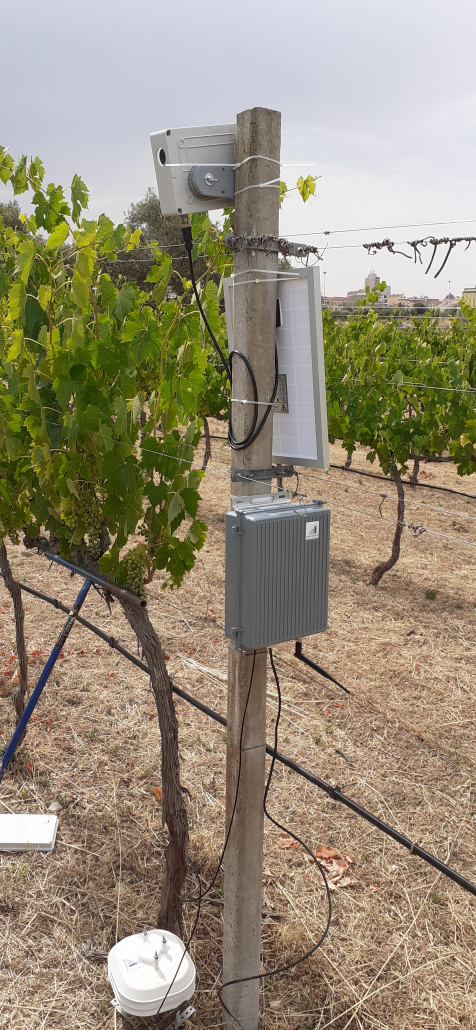
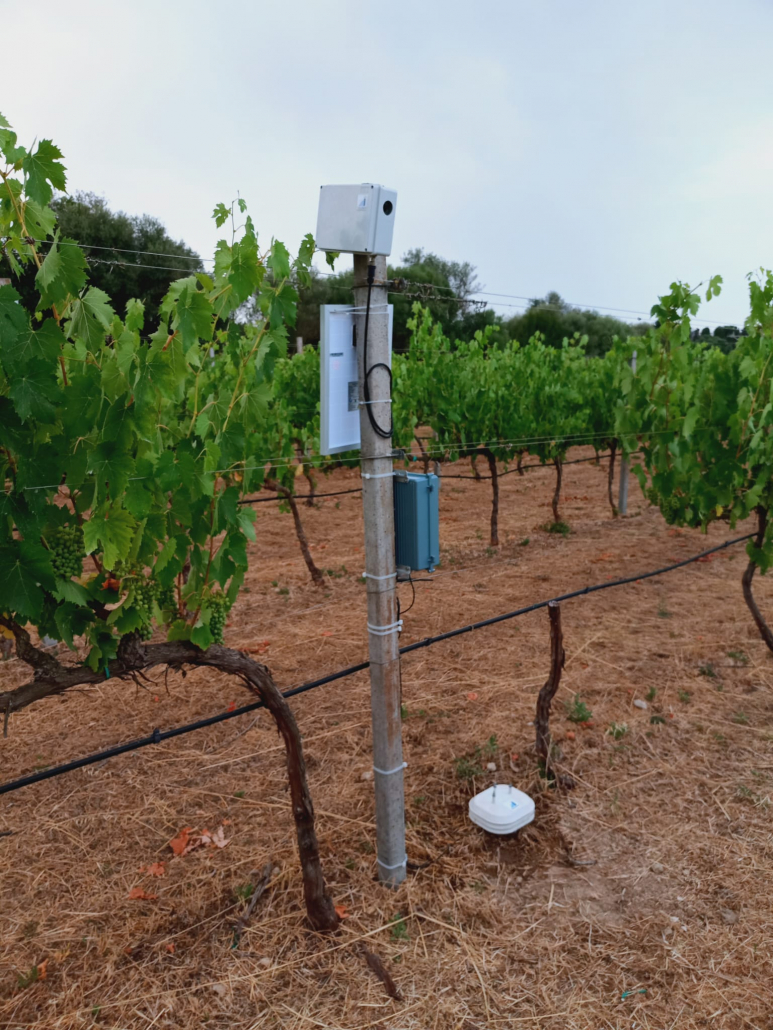
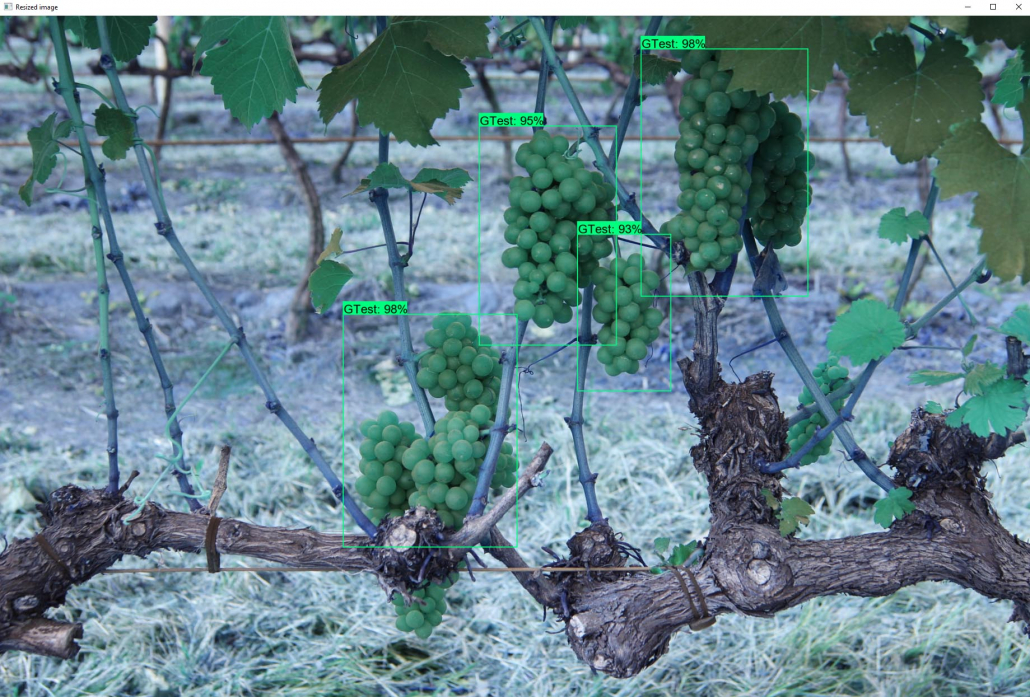
The goal of the project has been to design and develop a CPS solution according to the IoT/Predictive model paradigm. In this sense, the project has implemented a demonstrator of a innovative advanced system for management of downy mildew of the vine characterized by: innovative set of specific sensors (monitor with accuracy the hidro-termal conditions of oospores on the soil, monitor with high accuracy leaf wetness time-windows and the associated hidro-termal conditions, automatic monitoring and measurements of foliage/plant growth not covered by previous spraying) (KPI: run on plug-and-play battery for 3 years without any recharge and sending measured-data every 15 min) an effective predictive algorithm based on new parameters and a AI calibration system (KPI: accuracy higher than 90% with zero false-positive error). Another objective of the experiment is to keep production and service costs low in order to offer an affordable solution to the market.
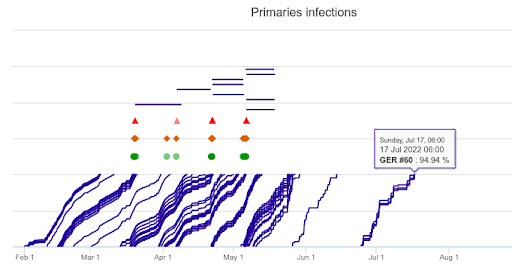